By Sam Linker
Introduction
In the film Moneyball, Jonah Hill’s Peter Brand tells Brad Pitt’s Billy Beane that his goal should be not to buy players, but to buy runs which served as the inspiration for this research project. The goal is to find an equation that includes a batter’s statistics as the variables in order to place a value on each stat in terms of runs. Also, I hope to provide a new perspective on evaluating players, and finding which areas should teams address to increase their chance of scoring more runs, and winning more games.
Data Collection
All my data was collected from fangraphs.com and all definitions for the statistics I used could be found in their glossary. I chose 16 statistics covering plate discipline, power, batted ball, and base running. They are the following:
BB%
K%
ISO
UBR
wGDP
wSB
LD%
GB%
FB%
Cent%
Oppo%
Soft%
Med%
Hard%
O-Contact%
Z-Contact%
I chose these 16 because I believe they represent different ways for ball clubs to improve their approach at the plate and on the base path. These data points along with runs scored were collected for every team from the 2002-2017 season. This resulted in 480 data points over the 16-year time span.
Data Analysis
I ran a regression using the 16 statistics as independent variables and runs scored as the dependent variable with the data. I chose to run all 16 at once because when judging a hitter and his contribution to runs scored, it is almost impossible to emphasize one variable while ignoring others. When teams decide to acquire a player, they look at the entire body of work, so that is what I will be doing. I then proceeded to do a backwards elimination on the data by eliminating the variable with the highest p-value. I repeated the regression with now 15 variables and continued this process until only variables with significant p-values of less than .05 remained. I ended up with a regression using 11 statistics of the original 16 which were the following:
BB%
K%
ISO
UBR
wGDP
LD%
GB%
Oppo%
Hard%
O-Contact%
Z-Contact%
The linear equation I calculated had an R2 value of .82 meaning 82% of the variability in runs scored could be explained by my model.
The equation to calculate runs scored is listed below.
Runs = -382.02 + 1685.51*BB% - 1407.53*K% + 2943.37*ISO + .78*UBR + 2.44*wGDP + 466.31*LD% + 388.08*GB% + 456.84*Oppo% + 385.31*Hard% + 75.48*O-Contact% + 278.96*Z-Contact%
To test how accurate this equation is, I took the 2017 team numbers from the 10 playoff teams, and placed them into the equation to get expected runs scored and compared that to actual runs scored for 2017. With the high R2 value and favorable results for the playoff teams, the model is an accurate predictor of runs scored when using the assigned data for the calculations.
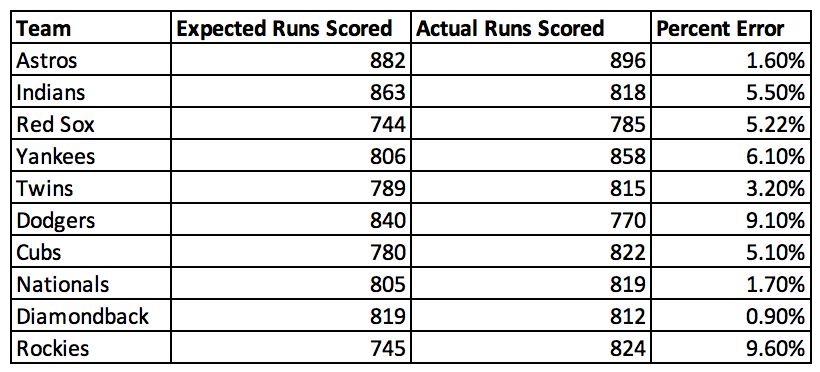
The predicted runs equation could also be used to identify how many runs are gained or lost by a 1% increase in one of the variable categories. UBR and wGDP go by an increase in .1 since those values are not measured on a percentage scale and typically range from -6 to 6 and -2.5 to 2.5 respectively. This change in measurement is indicated next to their names in the chart. The summary of this is found in the chart below.
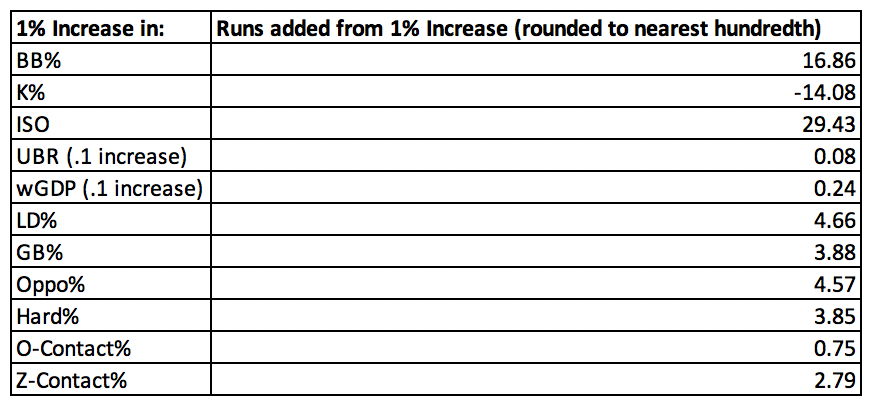
Conclusion
Using my regression model, I could come up with an equation that could serve as an accurate predictor for a team’s runs scored based off the sample and high R2. More importantly, I identified some new ways to add runs to a ball club. I knew coming into this project that BB%, K%, and ISO would generate the highest values for gaining or losing runs because of their direct correlation of getting runners on base and hitting for extra bases. A low value of UBR indicates that while smart base running does lead to more runs, it doesn’t suggest focusing on a player only for his base running skills. The batted ball data is the most interesting part of the results. Using the batted ball data, we can construct a perfect player who can immediately help your club by adding to your expected run total. The data shows that a line drive hitter who can hit the ball hard to the opposite field and make contact on most of the strikes he swings at is extremely valuable. If one player can help a team increase those percentages by 1% each, he can add 15.87 runs to his team, and that does not even factor in plate discipline, power, and base running. Overall, the results have the potential to help teams make better off-season and in-season decisions based on its effect on their expected runs by allowing teams to focus on where they need to improve and what type of player they should go after.
Comments